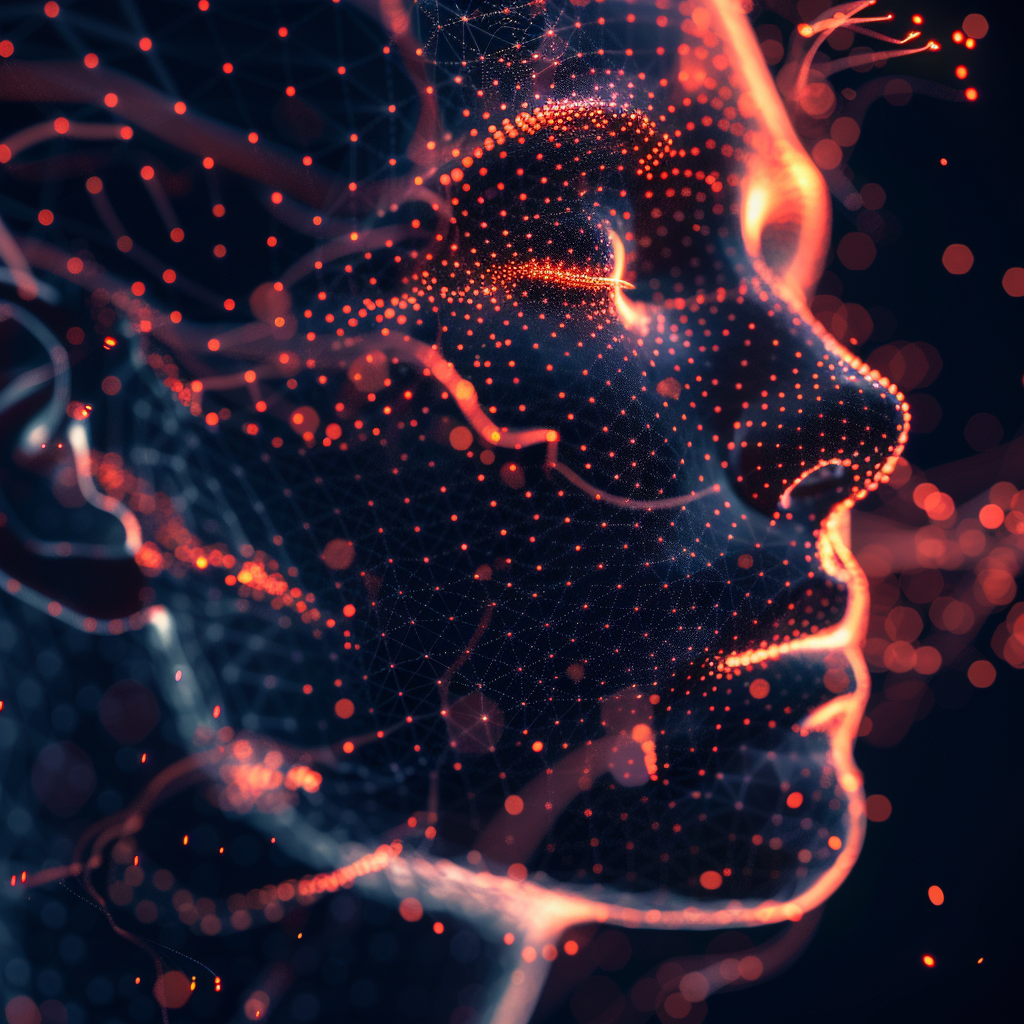
On July 12, 2024, the European Union officially published the AI Act in its Official Journal. The legislation will come into effect on August 1, 2024, 20 days after its publication. The implementation of this comprehensive regulation is structured in four distinct phases, spanning a three-year period:
- Phase 1 (February 2, 2025): The act will prohibit certain AI practices deemed to pose unacceptable risks.
- Phase 2 (August 2, 2025): Specific requirements for providers of general-purpose AI systems (GPAIs) will be enforced.
- Phase 3 (August 2, 2026): The majority of the regulation's provisions will take effect, including those pertaining to high-risk systems as outlined in Annex III.
- Phase 4 (August 2, 2027): The final phase addresses high-risk AI systems that are components of products already governed by existing EU harmonisation legislation.
This phased approach aims to provide stakeholders with adequate time to adapt to the new regulatory landscape while ensuring a comprehensive framework for AI governance across the European Union.
Data Governance Under the AI Act
Having high-quality, reliable data is crucial for effective decision-making in any organisation. Robust data governance is essential for ensuring valuable data's availability, integrity and security.
But data governance is not just about reducing the risks of misuse and manipulation, it’s also about supporting the democratisation of data, so the right people get the right data for the right causes.
Article 10
Data governance under the AI Act is particularly relevant in Article 10 of the regulation, which details strict data governance requirements for high-risk AI systems.
High-risk AI systems are defined by the general characteristics outlined in Article 6 of the regulation. In short, these are the systems that have the potential to cause significant harm to people’s safety, livelihoods, or fundamental rights.
They can either be AI systems used in safety-critical products, i.e. products already subject to other EU safety regulations (like toys, medical devices, and cars), or AI systems designed for specific high-risk applications, listed in Annex III of the regulation.
Requirements:
Training, validation, and testing data sets for high-risk AI systems must meet specific quality criteria (paragraphs 1 and 2).
These data sets must be:
- Relevant: aligned with the intended purpose of the AI system (paragraph 3).
- Sufficiently representative: reflect the real-world scenarios where the AI will operate (paragraph 3).
- Error-free (to the best extent possible): minimised errors to ensure reliable training and operation (paragraph 3).
- Complete: include enough information for the AI to function effectively (paragraph 3).
- Statistically sound: reflect the demographics impacted by the AI (paragraph 3, especially for personal data).
- Contextually relevant: consider the specific environment where the AI will be used (paragraph 4).
Data Governance and Management Practices:
Organisations developing high-risk AI systems must implement appropriate data governance practices (paragraph 2).
These practices address:
- Design choices: how the data will be used in the AI system.
- Data collection and origin: sources of data, especially for personal data (original purpose of collection).
- Data preparation: cleaning, labelling, and processing steps taken on the data.
- Assumptions behind the data: what the data represents and intends to measure.
- Data availability and suitability: ensuring there's enough appropriate data for the AI system.
- Bias detection and mitigation: identifying and addressing potential biases that could lead to unfair outcomes.
- Data gaps and shortcomings: identifying missing information and how to address it.
Special Considerations for Personal Data:
In exceptional cases, processing of special categories of personal data (e.g., race, health information) might be allowed for bias detection and correction (paragraph 5). This requires:
- Exhaustion of other options: Demonstrating that bias detection cannot be achieved with other data (e.g., anonymized data).
- Strict safeguards: Implementing technical limitations for data reuse, strong security measures, and access controls.
- Data minimization: Ensuring the special category data is only used for bias detection and deleted afterward.
- Transparency and accountability: Documenting why processing special categories of data was necessary and demonstrating efforts to minimise it.
High-Risk AI Systems Without Training:
For high-risk AI systems that don't rely on training data (e.g., knowledge-based systems), the data governance and quality requirements (paragraphs 2-5) only apply to the testing data sets (paragraph 6).